What is the best [Ai data collection for 2024]?
AI data refers to the vast amount of information collected, processed, and analyzed by artificial intelligence systems to learn patterns, make decisions, and improve performance. This data can come from various sources, such as sensors, databases, social media, and more, and is crucial for training AI models to understand and predict outcomes effectively.
AI Data Collection: A Comprehensive Guide
Introduction:
AI data collection is the cornerstone of building successful AI models. The quality, relevance, and diversity of the data directly influence how well an AI system performs. This guide will walk you through the essential aspects of AI data collection, ensuring that your data gathering processes are efficient, ethical, and effective.
1. Understanding AI Data Collection
AI data collection involves gathering and curating datasets that are used to train, validate, and test AI models. The data can come from various sources, including public datasets, surveys, sensors, and even synthetic data generated through simulations.
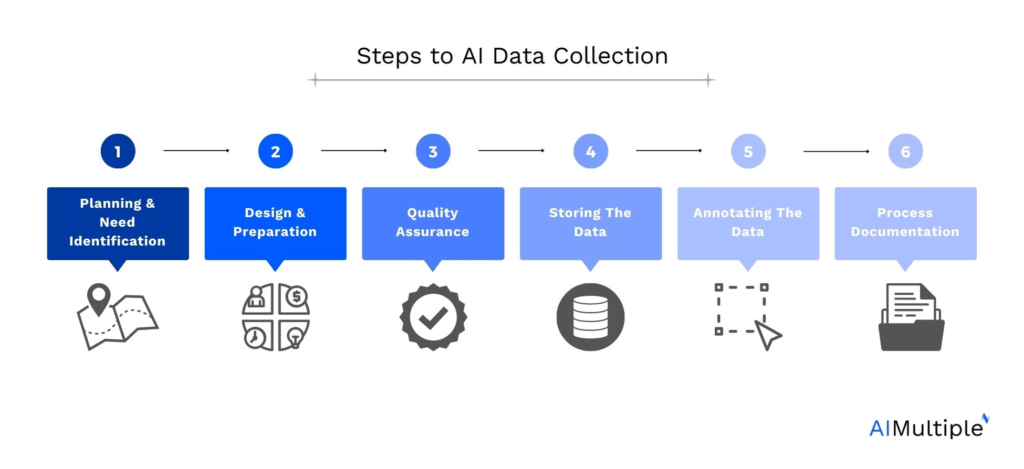
2. Importance of Data Quality
High-quality data is vital for AI model performance. Quality data is accurate, consistent, and free from errors. It should be well-labeled if used for supervised learning tasks, where the model learns from examples.

3. Data Relevance and Diversity
The data you collect must be relevant to the specific problem your AI model aims to solve. Additionally, the dataset should be diverse enough to represent various scenarios and minimize bias. This ensures that the AI system can generalize well across different situations.
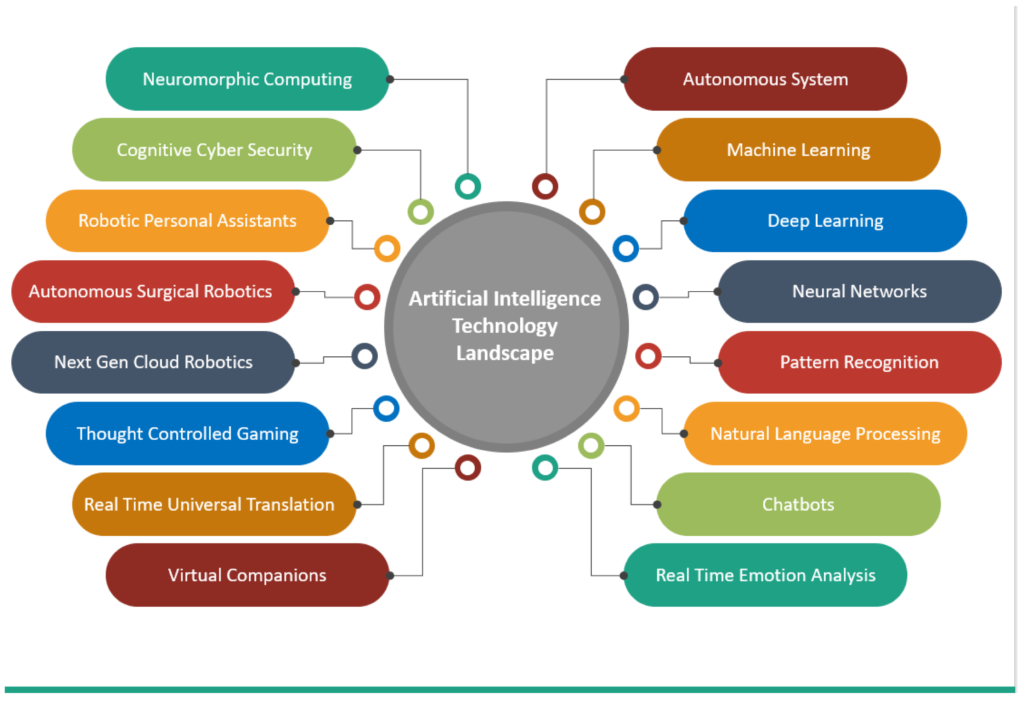
4. Ethical Considerations in Data Collection
Ethical considerations are crucial when collecting AI data. This includes respecting privacy, ensuring data is collected with consent, and mitigating biases within the dataset. Adhering to legal and ethical standards is not only a best practice but also helps build trust in AI systems.

5. Data Annotation and Preprocessing
Properly annotating data is essential for training supervised learning models. Annotations must be clear and consistent, aligned with the model’s objectives. Preprocessing steps like cleaning, normalization, and removing duplicates are also critical for ensuring the data is ready for model training.
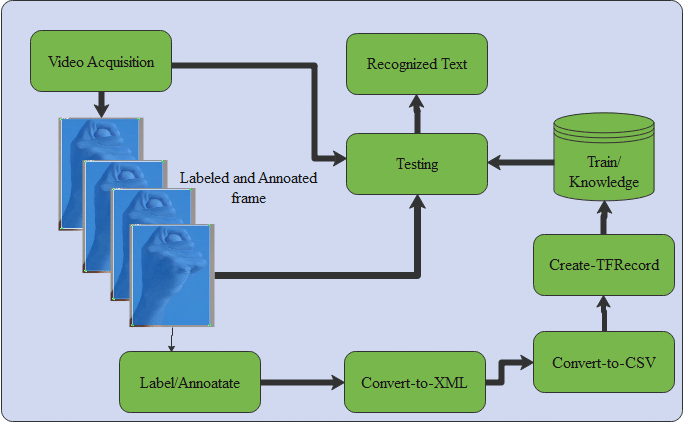
6. Continuous Data Collection and Updating
AI models benefit from continuous data collection, which allows them to adapt to new trends and changes in their environment. Regularly updating your dataset ensures that your AI model remains relevant and accurate over time.

.
Pros of AI Data Collection
- Efficiency and Automation: AI can process vast amounts of data quickly, automating repetitive tasks and freeing up human resources for more strategic work.
- Improved Decision-Making: AI-driven data analysis can uncover patterns and insights that might be missed by humans, leading to more informed and accurate decision-making.
- Personalization: AI data collection enables businesses to offer personalized experiences to customers by analyzing their preferences, behaviors, and interactions.
- Predictive Analytics: With AI, companies can anticipate trends and make proactive decisions based on predictive analytics derived from data.
- Scalability: AI systems can handle large volumes of data, allowing businesses to scale their operations without sacrificing performance or accuracy.
Cons of AI Data Collection
- Privacy Concerns: The collection and use of personal data raise significant privacy issues, potentially leading to misuse or unauthorized access.
- Bias and Fairness: AI systems can perpetuate or even exacerbate biases present in the data, leading to unfair or discriminatory outcomes.
- Data Quality Issues: Poor quality or incomplete data can lead to inaccurate AI predictions and decisions, undermining trust in the system.
- High Costs: Implementing AI data collection and processing systems can be expensive, requiring significant investment in technology and expertise.
- Ethical Considerations: The ethical implications of AI data collection, such as surveillance and the potential loss of jobs due to automation, are complex and require careful consideration.
Conclusion
Effective AI data collection is a multi-step process that requires careful planning and execution. By focusing on data quality, relevance, diversity, and ethics, you can build AI models that perform well and are trustworthy. Whether you’re working with publicly available datasets or collecting custom data, these best practices will guide you in building a solid foundation for your AI projects.